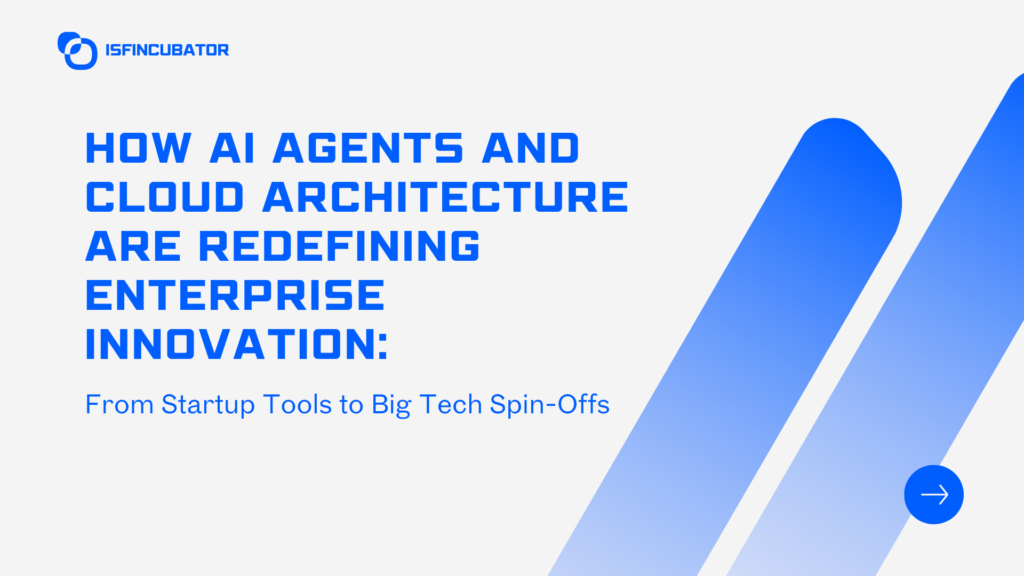
AI Agents in the Cloud: Data Architecture Meets Automation
As businesses increasingly adopt AI agents, the conversation is quickly shifting from simple task automation to how these agents interact with cloud infrastructure and modern data architectures. And this isn’t just for tech giants – it’s becoming a foundational strategy for startups looking to scale efficiently.
At the heart of this movement is a simple truth: AI agents are only as good as the data they can access. That’s why forward-thinking companies are investing heavily in cloud-native data platforms—such as Snowflake, Databricks, or Google BigQuery – and designing architectures where structured and unstructured data is accessible, queryable, and secure. In such setups, AI agents don’t just perform pre-scripted tasks – they analyze, summarize, trigger workflows, and even generate new business logic based on real-time data.
Take Notion AI for example. They embedded a generative AI layer directly into their workspace product – meaning the agent not only pulls from the user’s workspace but also learns from context. This required a cloud-based, vectorized storage layer capable of ingesting, indexing, and recalling text across projects, meetings, and notes. Their success lies not only in the AI interface but in the infrastructure behind it.
Or consider Linear, a project management platform that deployed an internal AI agent trained on their own data schema. It summarizes team progress, generates updates, and even opens tickets. Under the hood? An event-driven architecture leveraging Kafka and a unified warehouse that syncs across the entire development stack.
Here’s a simplified flow of how these systems typically work:
User Request → AI Agent Layer → Query Engine (e.g. Big Query) → Data Lakehouse or Warehouse → Business Logic/API Layer → Output / Trigger
- AI Agent Integration with Cloud Infrastructure
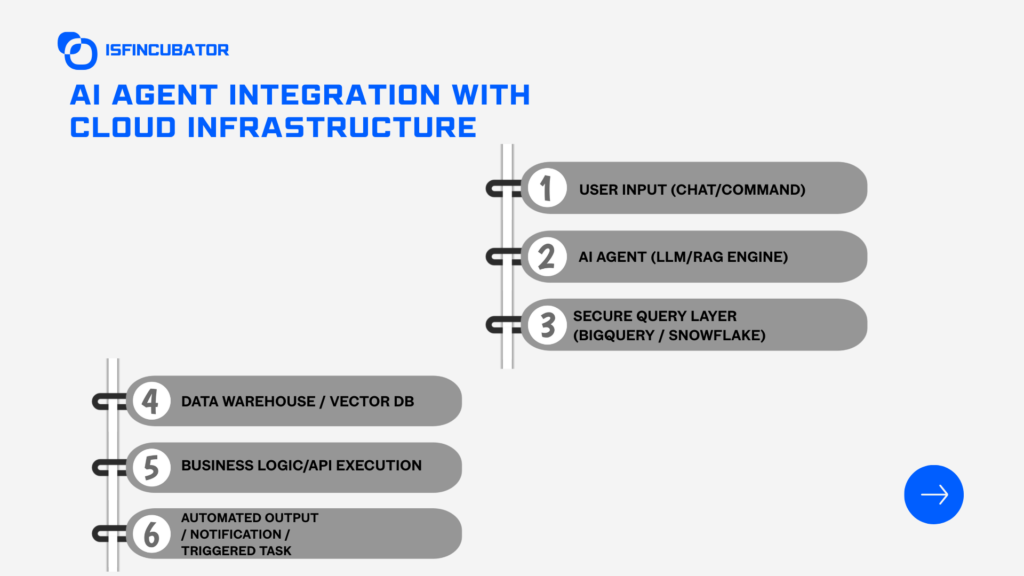
- User Input (Chat/Command)
↓ - AI Agent (LLM/RAG Engine)
↓ - Secure Query Layer (BigQuery / Snowflake)
↓ - Data Warehouse / Vector DB
↓ - Business Logic/API Execution
↓ - Automated Output / Notification / Triggered Task
In startups with tighter resource constraints, this flow is often containerized using lightweight Kubernetes clusters and serverless compute tools (e.g. Google Cloud Run, AWS Lambda), reducing infrastructure overhead.
Uprising Startups Powering Global Enterprises
More importantly, a number of once-small startups are now supplying these AI agent infrastructures to major corporations:
- Cohere began as a niche NLP model provider and now powers custom LLM solutions inside Fortune 500 data stacks, often embedded within private infrastructure for compliance.
- Glean, founded by ex-Google engineers, offers AI-powered enterprise search across SaaS tools and internal databases. Today, they support data unification at scale for firms like Airtable and Canva.
- Upstage, a Korean AI startup, developed a GPT-based enterprise document agent that is now being used by government and manufacturing clients in Southeast Asia. Their agent is optimized for private RAG pipelines over internal knowledge bases.
These companies succeeded by solving both the infrastructure side (connectors, indexing, real-time ingestion) and the usability layer (chat-style UI, integration with Slack or Teams, secure auth).
Some of these companies are already seeing significant revenue:
- Glean raised $100M+ at a valuation over $1B, with ARR reportedly exceeding $25M.
- Upstage, though younger, closed major B2B contracts with Korean conglomerates and is now expanding into multilingual document analysis for Japanese and Taiwanese enterprise use.
Spin-off Success: When In-House Innovation Turns Into Real Business
Enterprise-backed internal venture programs have also fueled the rise of AI-driven solutions. Take Samsung’s C-Lab, which began as an internal incubation system but has spun off dozens of independent startups. Among them:
- Nota AI: Originally developed within Samsung’s internal labs, Nota specializes in model compression and edge deployment for AI. Since spinning off, it has secured major clients in both Korea and Europe.
- Plask: A motion capture startup born from C-Lab, now works with animation studios in Japan and the U.S. by providing AI-driven mocap tools through a lightweight SaaS.
From the U.S., Google’s Area 120, an experimental incubator has similarly launched AI-driven tools and platforms. One of its most successful spin-offs, Tables, began as an internal automation solution for structured workflows and was later integrated into Google Cloud services.
Other Area 120 graduates like Stacker (a no-code business app builder with AI assistance) and ThreadIt (a video messaging platform with auto-captioning and summarization) were either acquired by other teams internally or spun into public offerings. These illustrate how large tech firms experiment, validate, and sometimes commercialize AI-agent-inspired tools internally before pushing them to market.
Big Tech’s Strategic Bets on AI Agents
The rise of autonomous AI agents hasn’t gone unnoticed by the industry’s largest players. Google, Meta, and AWS have all aggressively invested in AI-agent-enabling infrastructure and startups:
- Google has launched Gemini 1.5 Pro and Flash, explicitly designed to power real-time AI agent actions, document parsing, and multi-modal reasoning. They’ve also backed startups like Reka AI, which builds multi-agent orchestration frameworks.
- Meta introduced its AI Studio, empowering developers to build personal AI agents tied to Meta platforms like WhatsApp, Messenger, and Instagram. Their LLaMA models are also being tuned for on-device agents.
- AWS invested heavily in Anthropic, the creator of Claude, which is now integrated into Amazon Bedrock for secure enterprise use cases. AWS also enables startups to build RAG-style agents using its serverless ecosystem and proprietary vector databases.
These efforts show that AI agents aren’t just trends. they’re forming the next layer of the cloud-native stack. Investments aren’t only technical, they’re strategic bets on how humans and machines will collaborate across every tool, platform, and enterprise.
Together, these initiatives signal one thing clearly: AI agents are no longer optional -they’re foundational to modern digital business.
These spin-offs and internal transitions show how major tech firms are not only incubating innovation but strategically integrating or commercializing AI agents and technologies—whether through internal scaling or market-facing platforms.
Enterprise-backed internal venture programs have also fueled the rise of AI-driven solutions. Below are notable spin-off cases from global tech giants:
- AdLingo (Google): Originally incubated within Area 120, AdLingo built a conversational marketing platform enabling brands to launch AI-powered interactive ads. It was later acquired by Google’s parent company Alphabet and folded into the broader Ads division, contributing to customer engagement tools.
- FindTime (Microsoft): Developed within Microsoft Garage, FindTime started as an internal AI-powered meeting scheduling assistant. It gained traction across Microsoft’s productivity teams and is now fully integrated into Microsoft Outlook and Microsoft 365.
- Einstein GPT Features (Salesforce): Salesforce Labs regularly prototypes AI capabilities such as predictive lead scoring and intelligent workflow recommendations. Several of these features—like Einstein Opportunity Insights—originated internally and evolved into paid product lines used by thousands of Salesforce clients.
- Alexa Smart Scheduling (Amazon): Amazon’s Grand Challenge program produced an AI-based smart scheduling and routing system initially developed for internal logistics optimization. It now supports Alexa’s proactive features and is partially embedded in AWS for enterprise delivery clients.
These cases show that companies like Google, Microsoft, Salesforce, and Amazon are not only investing in AI research, but also operationalizing it through internal innovation programs that can evolve into monetized, real-world products., which began as an internal incubation system but has spun off dozens of independent startups.
This growing synergy between cloud-native infrastructure and AI agents isn’t just about tech sophistication – it’s a competitive differentiator. Startups that treat AI agents as extensions of their infrastructure, not just plugins, are the ones setting new standards for responsiveness, personalization, and automation.
Whether you’re running a three-person product team or a 300-person tech company, the message is clear: If your data is ready, your AI agent can go from helpful to game-changing.