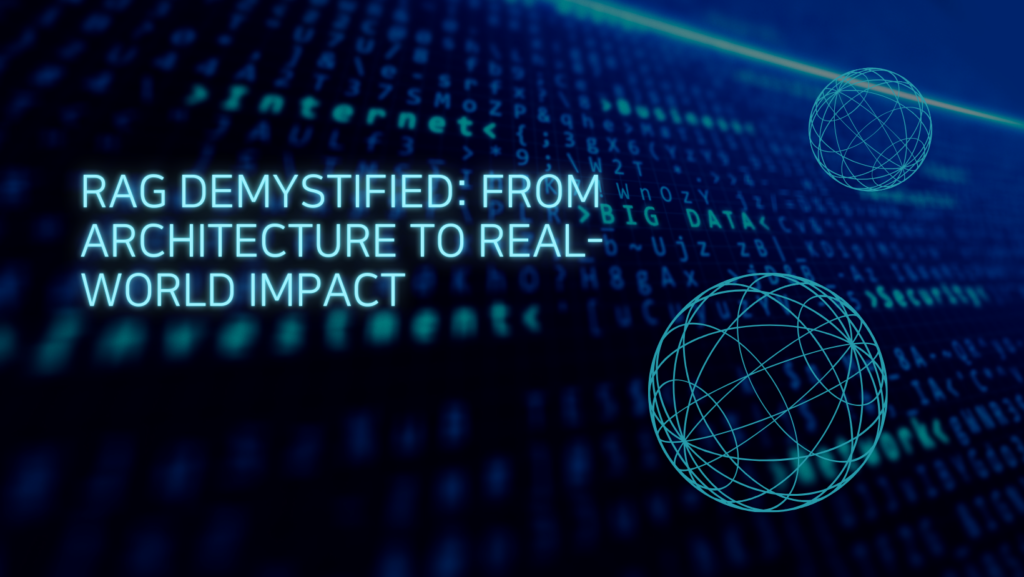
Index
-What Exactly Is RAG?
-Under the Hood: How the RAG Process Actually Works
-Why RAG Isn’t Just Technical. It’s Practical
-RAG’s Value in a Business Context
-The Technology Stack That Powers RAG
-Challenges and Limitations of RAG
-When to Use RAG and When Not To
-Security and Privacy Considerations
-What Comes After RAG? Emerging Directions
RAG: Beyond Generation: How Retrieval Augmented AI Is Reshaping the Way We Work
If you’ve ever been blown away by an AI like GPT-4 or Claude smoothly answering a tricky question, you’re not alone. Whether it’s drafting emails, writing code, or summarizing long documents, these tools are undeniably impressive. But let’s be honest they’re not perfect. Every now and then, they’ll say something that sounds smart… but just isn’t true. That’s what we call a hallucination, and in real-world business settings, getting facts wrong can be a pretty big deal.
That’s where something called RAG short for Retrieval-Augmented Generation comes in. RAG is kind of like giving your AI a library card. Instead of making stuff up from memory, it checks the facts first. It connects the language model to up-to-date info before answering your question. So rather than relying solely on what the model remembers, it pulls in the latest, most relevant context then gives you a response that’s not only well-written but also accurate.
What Exactly Is RAG?
Let’s say your AI is like a really smart coworker who read everything up to 2023, but hasn’t kept up since. If you ask them about a policy that changed last month or a project update from last week, they’ll try to answer. But without new info, they might miss the mark.
With RAG, that coworker gets access to the latest internal docs, reports, or wikis before speaking up. It’s like saying, “Hey, take a second to read this page first.” The result? More helpful, reliable answers and fewer awkward, inaccurate ones.
So when a RAG-powered AI gives you an answer, it’s using real data, not just guesswork. That means you can trust it more and maybe even use it for things that really matter.
Under the Hood: How the RAG Process Actually Works
So how does RAG actually work? Here’s a simplified breakdown:
First, someone asks a question. Maybe it’s “How do we handle refunds for premium users?” That question gets turned into a mathematical representation called a vector using an embedding model. Vectors help the AI understand the meaning of the question.
Next, that vector is used to search a special kind of database called a vector database that holds your documents in vector form. It digs through thousands of files and picks out the most relevant ones.
Those selected documents get bundled up and handed off to the AI model like giving someone the right pages in a binder before they answer a question.
The model reads through those documents and generates a response based on what it just learned. So the answer you get isn’t just based on what the model knows it’s grounded in real, current data.
One of the best parts? You don’t have to retrain the whole model every time something changes. Just update the documents, and your AI assistant will stay up to date.
Why RAG Isn’t Just Technical – It’s Practical
You don’t need to be an AI expert to appreciate what makes RAG so useful. Think about how often you or your team dig through emails, Slack, Notion, or PDFs just to find one answer. Now imagine typing your question into a chat box and getting that answer instantly, and accurately.
That’s what RAG enables. It’s already being used in the real world by all kinds of teams. For example, a product manager can ask, “What did we ship in Q1?” and get a response pulled directly from the changelog and product roadmap.
Support agents don’t have to dig through help docs RAG reads those for them and gives real-time, customized responses. Legal teams can search for the latest contract language, and researchers can get summaries from relevant studies all with less effort.
Even engineering teams use it to scan codebases and documentation to suggest changes or explain existing systems. Basically, anywhere people search for answers, RAG can make their lives easier.
It’s not just smart it’s helpful.
The Technology Stack That Powers RAG
RAG might sound like a fancy concept but setting it up isn’t as hard as it sounds especially with the growing set of tools out there.
First, you’ll need an embedding model to turn your text and questions into vectors. You can get this from OpenAI, Hugging Face, Cohere, and others.
Next, you’ll need a vector database to store and search for those embeddings. FAISS is a solid open-source option, while Pinecone and Weaviate offer managed cloud services with advanced features.
For the actual response generation, you’ve got your pick of LLMs: GPT-4, Claude, Mistral, LLaMA 3 the list keeps growing. These models take the retrieved info and turn it into polished answers.
And to tie it all together, frameworks like LangChain, LlamaIndex, and Haystack help orchestrate everything smoothly.
Even better, you can plug in your own data throughout the process meaning the system doesn’t just sound smart, it actually knows your business.
RAG’s Value in a Business Context
Here’s the big question: is RAG just another cool demo, or is it actually useful at work? The answer is: very useful.
When companies add RAG to their stack, they unlock real productivity. Teams find information faster, onboard more quickly, and spend less time repeating questions. And because the answers come with context, there’s less back-and-forth and more action.
From a cost perspective, it’s a win too. You don’t need to constantly fine-tune your model or rebuild systems every time something changes. Just update your internal docs, and the system keeps up.
Companies like Upstage are already helping organizations roll out full RAG pipelines, and platforms like Glean and You.com are baking RAG directly into their user interfaces.
In short, RAG brings AI from the lab to your desk and makes it practical.
Challenges and Limitations of RAG
That said, no system is perfect. RAG has a few challenges to be aware of.
First, retrieval quality really matters. If your vector database pulls the wrong documents, even the best language model can get confused. You need clean, well-organized content and solid search logic.
There’s also the issue of token limits. Language models can only read so much text at once, so you can’t just dump in dozens of documents and expect a perfect answer. You’ll need to filter and prioritize what the AI sees.
And don’t forget latency. Adding a retrieval step can slow things down, especially if your stack relies on multiple external services. Optimization is key for keeping response times low.
When to Use RAG – and When Not To
RAG isn’t a silver bullet for everything. It works best when your goal is to answer questions based on factual information like internal policies, legal clauses, customer support flows, or technical documentation.
If you’re doing creative writing, long-form brainstorming, or abstract problem-solving, sometimes a plain language model works just fine and might be faster.
RAG also works best when your knowledge base is well maintained. If your data is scattered, outdated, or poorly labeled, you’ll likely get inconsistent results.
The key is to match the tool to the task.
Security and Privacy Considerations
Bringing company data into any AI system always raises important questions about security and compliance.
With RAG, you’ll want to ensure that retrieval respects user permissions meaning the AI only sees what the user is allowed to see. Logging, access controls, and audits all matter here.
For sensitive environments, running everything on-premises or in a private cloud is often the way to go. Open-source tools like FAISS and LangChain make this feasible for most teams.
Don’t skip this step, trust is everything.
What Comes After RAG? Emerging Directions
RAG is powerful, but it’s also just the beginning. The next wave of innovation is already taking shape.
We’re seeing multi-agent systems where one AI retrieves info, other reasons about it, and a third generates output. This lets each component specialize and can lead to smarter results.
There’s also growing interest in feedback loops, where user corrections are fed back into the system to improve future answers. Imagine an AI that learns not just from training data, but from how you actually use it.
And researchers are even combining RAG with logic-based systems to create hybrid models that not only look up facts but also think through them.
It’s an exciting time and if RAG is step one, what comes next is going to be even more transformative.